HAWK4Label: HumAn-enhanced WeaK supervised learning for data LABELing in the depalletization process
Part of the project AI REDGIO 5.0 | EU Horizon Europe
(Grant Agreement N. 101092069)
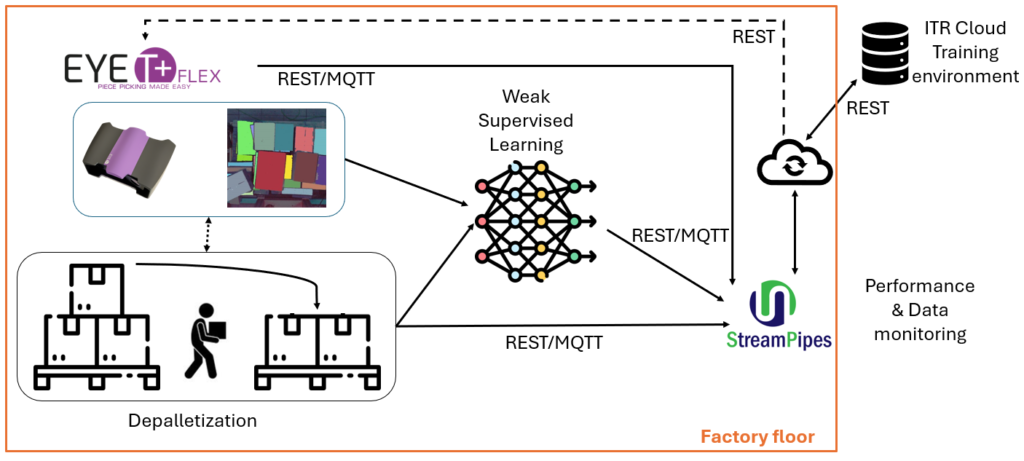
CHALLENGES
In Machine Learning (ML), the critical task of data labeling poses significant challenges, particularly in terms of data quality, domain discrepancies, overfitting, and the intensive effort required for accurate annotation.
This is significantly pronounced in industrial contexts, where existing datasets often fail to represent real-world complexities, leading to models that struggle with generalization and adaptation to new scenarios.
BENEFIT
This methodology promises to revolutionize the labeling process by making it more efficient, achievable, cost-effective, and human-centric. It reduces the programming effort traditionally required for each specific object or scenario, as models can adapt to new tasks with minimal additional input. It also offers superior object recognition and handling, even when objects are randomly oriented or closely packed, facilitating more effective bin-picking and depalletizing operations.
Furthermore, the solution is scalable, allowing for easy adaptation and retraining as new objects or tasks are introduced, ensuring that the system evolves alongside industrial needs. This technology can simplify and accelerate the deployment, allowing even non-skilled operators to train the system and enabling a wide adoption with very different objects and sectors, this is another crucial element that rises from the human-centric approach adopted in the proposed HAWK4Label system. The system will dynamically adapt to market needs and customize production according to specific requirements. This allows for more flexible and tailor-made production to meet customers’ needs and adaptation to multiple and different industrial sectors.
APPROACH
HAWK4Label is an innovative solution for image data labeling. It will exploit a weak-supervised deep learning approach for auto-labeling mixed with the human’s feedback to improve the labeling. The vision system will be designed and implemented to learn from the interactions and experiences of human workers. This fosters a collaborative learning environment where machines and human workers can share knowledge and skills to improve production quality and efficiency.
The operator can provide feedback to the AI system for learning and improvement.
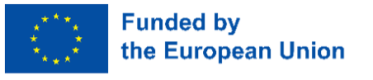
Funded by the European Union. Views and opinions expressed are however those of the author(s) only and do not necessarily reflect those of the European Union or Health and Digital Executive Agency (HaDEA). Neither the European Union nor HaDEA can be held responsible for them.